COVID-19 has revealed the many shortcomings of modern healthcare delivery, ranging from widespread patient overflow to poor infection control protocols, particularly failing poor communities and those of color.
The longer patients stay in the hospital, the more likely they will suffer from preventable injuries. Hospitals spend $2 billion on avoidable hospital-acquired conditions like pressure sores, falls, and infections every year. Avoidable complications cause patients to stay on average eight more days, and their likelihood of death increases by 72%.
Even before COVID-19, burnout rates among nurses reached 40%, increasingly due to growing pressure on nurses from the growth in the number of patients with more extended hospital stays. Currently, hospitals spend upwards of $6.9 million per year in turnover and recruiting costs.
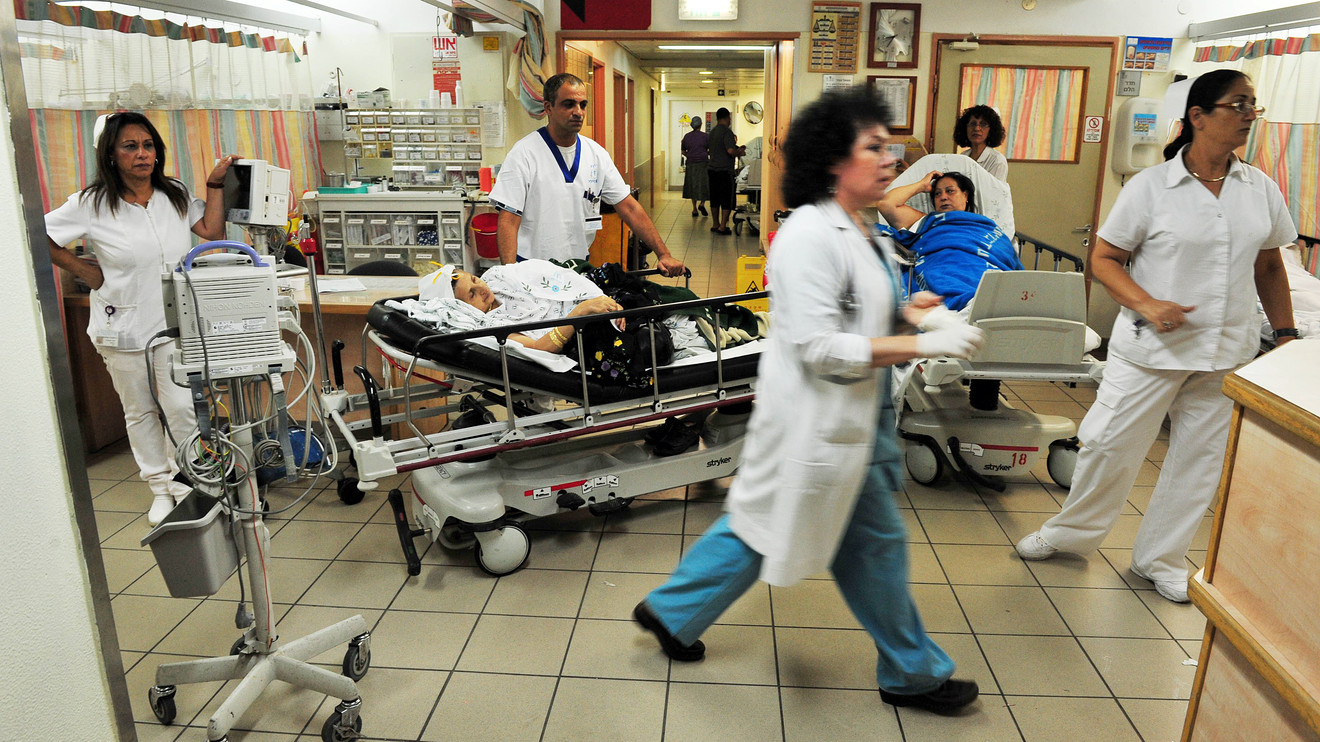
The cost of healthcare is a significant barrier to access for many individuals. Without a way to reduce staff burnout and decrease operational hazards that increase costs, healthcare remains unaffordable.
A new paradigm in information technology, Ambient Intelligence (AmI), holds the potential to transform healthcare for the better. It can be embedded into the physical environment, supporting hospital staff and patients at every step of the way. Ambient intelligence can assist with clinical decision-making, reduce staff burnout, decrease costs, and improve patient safety. By relieving healthcare workers of documentative and repetitive tasks, ambient intelligence improves clinical workflow, overall improving the quality of healthcare delivery and productivity.
How Ambient Intelligence Works
Unobtrusive, contactless sensors embedded in the environment allow physical spaces to be sensitive and responsive to patient movement. These sensors create a ubiquitous digital environment that is aware of peoples' presence, context-aware, adaptive to patients' continuing health needs, personalized, anticipatory, and transparent.
Ambient intelligence gathers data on patients in real-time, gives clinicians helpful insights on the everyday behavior of patients, prevents patient deterioration, and allows more independence for patients, faster recovery time.
Decision support systems can assist clinicians in making the best diagnostic and treatment options possible. These systems assist clinicians in maintaining people-centered approaches in otherwise metaphorically dark spaces by using context information, goal recognition, and case-based reasoning.
Early Rehabilitation with Continuous Monitoring
By monitoring behavioral signs in patients, ambient intelligence can predict and prevent medical emergencies.
Behavioral monitoring is instrumental in postoperative care, where for example, increasing patient mobility reduces time to recovery. According to a study by Corcoran et al.5, increasing mobility by approximately 1 hour per patient decreased length of stay by almost 40%.

By tracking patient mobility in postoperative care environments, nurses and physical therapists can understand how active a patient is, see their trend compared to the rest, and intervene early before the patient deteriorates. They can show patients how much they have been moving over the day and motivate them to increase their mobility, allowing them to recover faster.
Reducing ICU Stay
In the United States, ICUs cost the health system $108 billion per year, making up 16% of all hospital costs. Furthermore, ICU-acquired ailments are potentially responsible for doubling the yearly mortality rate. Ambient sensors provide the continuous and nuanced understanding needed for the early mobilization of patients in the ICUs. These sensors can detect external assistance or interactions with the physical environment.
Nurses can use AmI insights to detect delirium in patients early. It also can provide a deeper understanding of the correlation between patient mobilization and patient recovery. For example, researchers found that sensors detected significantly fewer head movements between ICU patients with delirium.
Keeping Elderly Safe
In nursing homes, any changes in activities may be an indicator of cognitive or physical decline.
According to a survey on ambient intelligence in health care, researchers identified changes in movement patterns, walking speed, number of outgoings, and sleep rhythm have as early signs of dementia. Ambient technology can allow individuals with disabilities to maintain a more independent lifestyle using home automation, perform continuous cognitive and physical monitoring, and provide them with real-time assistance. For example, ambient systems can remind a forgetful elderly patient to take their medicine.
Falls are also a critical problem for elderly individuals. Ambient sensors such as passive infrared (PIR) and pressure sensors prevent falls or use floor vibration detection and ambient audio analysis to detect when a fall happens. Visual systems can use 3D motion, shape, and inactivity to prevent or detect falls.
Ethical Ambient Intelligence
For ambient intelligence to be a welcome tool in the clinical world, it must ensure data privacy and model transparency. One privacy-preserving technique is to de-identify data by removing the identities of individuals. Another method is data minimization, which reduces data capture by pausing the ambient system when a patient is out of the room.
The implementation of ambient intelligence requires the cooperation of healthcare professionals, researchers, and computer scientists with law, ethics, and public policy experts to ensure the creation of fair and trustworthy systems.
At Ouva, we address concerns surrounding data privacy by processing any visual information in real-time to store no recognizable image. Additionally, Ouva depersonalizes identifying people by markers like clothing rather than using facial recognition. We work with healthcare systems to run the Ouva platform on-premises if needed so that no data leaves the hospital's walls.
For Artificial Intelligence-based AmI solutions to perform equitably for everybody, the data they learn from must be diverse in many aspects. The result of using a biased system can vary from making a wrong categorization in a patient's age to a deadly fall that goes undetected. For example, Larrazabal et al. showed that an X-ray algorithm trained with male data could not read female X-rays well. Data diversity in AI is so crucial that Moderna delayed COVID-19 vaccine trials to ensure minority representation in the middle of the pandemic.
Better Healthcare with Ambient Intelligence
Not only do ambient intelligence systems make hospitals safer, but they make healthcare more affordable by allowing staff to care for patients as freely and conveniently as possible. Based on the USA's Affordable Care Act value-based model, hospitals with higher quality care can lower tax liabilities, and those with low scores are penalized. According to Healthpayer Intelligence, the Affordable Care Act rewards providers "for the quality of care they deliver as a part of the quadruple aim: improved patient care, improved population health, lower healthcare costs, and increased provider satisfaction." Healthcare systems that recognize this potential are likely to benefit from implementing Ambient Intelligence in their care environments.
With the future of healthcare at stake, ambient intelligence is the way to ensure equitable care delivery to all.
- IBM. (n.d.). Hospital-acquired conditions lead to avoidable cost ... - IBM. Retrieved November 22, 2021, from https://www.ibm.com/downloads/cas/X97QXLER.
- Priscilla Holdren et al. “Burnout syndrome in hospital nurses”, Marshall University
- 2019 National Healthcare Retention & RN Staffing Report, Nursing Solutions, Inc.
- Corcoran et al. Early Rehabilitation in the Medical and Surgical Intensive Care Units for Patients With and Without Mechanical Ventilation. PM R. 2017 Feb;9(2):113-119. doi: 10.1016/j.pmrj.2016.06.015. Epub 2016 Jun 23. PMID: 27346093.
- Davoudi, A., Malhotra, K.R., Shickel, B. et al. Intelligent ICU for Autonomous Patient Monitoring Using Pervasive Sensing and Deep Learning.
- G. Acampora, D. J. Cook, P. Rashidi and A. V. Vasilakos, "A Survey on Ambient Intelligence in Healthcare," in Proceedings of the IEEE, vol. 101, no. 12, pp. 2470-2494, Dec. 2013, doi: 10.1109/JPROC.2013.2262913.
- Agostina J. Larrazabal, Nicolás Nieto, Victoria Peterson, Diego H. Milone, Enzo Ferrante, "Gender imbalance in medical imaging datasets produces biased classifiers for computer-aided diagnosis"
- Meg Tirrell, Leanne Miller, "Moderna slows coronavirus vaccine trial enrollment to ensure minority representation, CEO says"
- The Defining Features of Current Value-Based Care Models, Healthpayer Intelligence