The longer patients stay in the hospital, the more likely they will suffer from preventable injuries. Hospitals spend $2 billion on avoidable hospital-acquired conditions like pressure sores, falls, and infections every year. Avoidable complications cause patients to stay on average eight more days, and their likelihood of death increases by 72%.
World Health Organization data clearly shows the growing need for a new way in healthcare, especially in postoperative care: Global population aged over 60 is on the path to increase by 22% from 2015 to 20502. The growth in the number of patients with more extended hospital stays is increasing pressure on nurses. Even before COVID-19, burnout rates among nurses were reaching 40%. Currently, hospitals spend upwards of $6.9 million in turnover and recruiting costs annually.
Radboud University Medical Center (Radboudumc) in the Netherlands, where the elderly age group will reach a quarter of the population by 2050, has certainly felt the urgency. The number of people working in healthcare should dramatically increase in the coming decades, or a significant technology innovation needs to happen in how hospitals deliver care.
Before implementing Ouva, researchers from Radboudumc started a worldwide initiative called R4Heal, to reduce hospitalization, readmissions, and length-of-stay by creating more intelligent patient rooms. Researchers have discovered that the more patients recover uneventfully, the quicker they get better. In particular, they have found that mobility, sleep, pain, and stress all contribute to a patient's recovery. In the center of this transformation lies a data-centric operation.
Radboudumc sought an intelligent and customizable technology to make this transformation and considered other local and global companies. They realized they could not achieve their aims without reliable data and an AI platform to uncover actionable insights. Over a carefully designed validation & evaluation process, Ouva's responsibilities went from fall prevention alone to preventing patient deterioration, increasing nurse capacity, and more.
Multi-dimensional Collaborative Implementation
Radboudumc rigorously tested the efficacy of Ouva in multiple dimensions. The research team analyzed the accuracy of every metric and the user experience over a year-long study.
Twenty-seven patients at Radboudumc volunteered to participate in the study. The research team observed and compared the activities performed by each patient and compared them to the data generated by Ouva to understand the accuracy.
“With real-time metrics and alerts made available by Ouva, our staff and management can identify the root causes of our challenges and delays and create accurate course corrections.” — Prof. Dr. Harry van Goor
The implementation team at the hospital designed the process to ensure the hospital staff embraced Ouva rather than being another tool they will eventually discard. To accomplish this, nine surgery and ICU nurses, nurse researchers, physicians, and interaction designers used Ouva and provided extensive feedback about how it works in daily care.
The Radboudumc staff found Ouva very useful for daily care. Out of all, they found the ability to track patients' in-room activity to be the most impactful. All interviewees unanimously acknowledged that the live view brings clear visibility to all patients on the floor - the ability lacking in the current care process. Through a collaborative process, the Ouva team quickly received and implemented their additional recommendations into the product today.
Based on the comprehensive validation, Radboudumc is rolling out Ouva AI for postoperative care and has set a clear roadmap for Ouva to be Radboudumc's AI partner throughout their transformation of all their surgical departments.
During the study, Ouva demonstrated its capabilities in three main areas.
Early Rehabilitation with Mobility Tracking
Staying on top of mobility is one of the most critical steps towards reducing length-of-stay. With Ouva's mobility tracking, the nurses at Radboudumc understand how active a patient is, see their trend and intervene early before the patient deteriorates.
.png)
Preventing Avoidable Injuries
Non-fatal fall injuries cost US hospitals $50 billion per year and $754 million on fatal falls. Ouva can notify nurses in Radboudumc the moment the patient sits up, a significant 30-to-60 second improvement towards fall prevention compared to bed sensors that alert when a patient gets out of the bed.
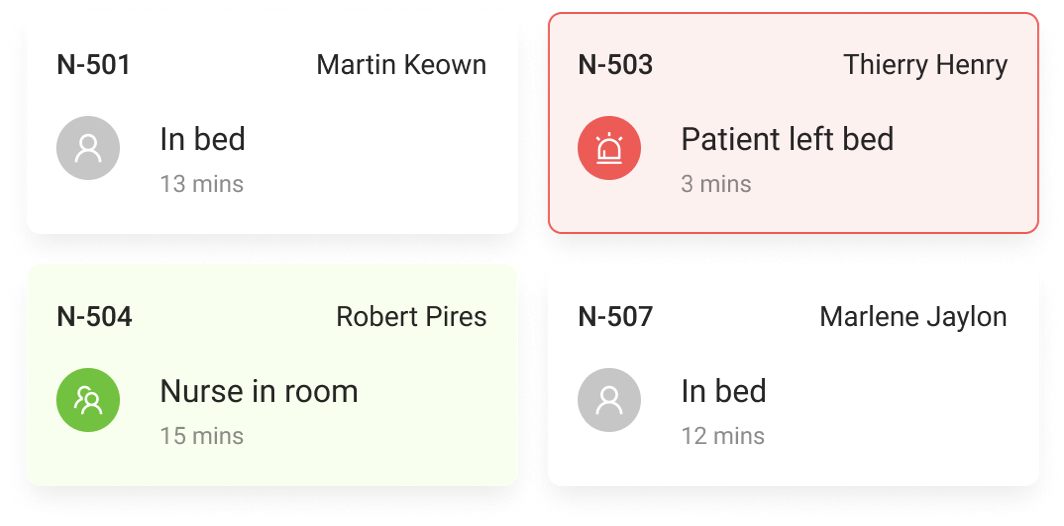
Increasing Nurse Availability
Pennsylvania researchers discovered that they could prevent 4,160 infections by reducing burnt-out nurses from 30% to 10%. With Ouva's nurse visit analysis module, administrative staff can plan capacity well ahead because they precisely know what types of patients require how much nurse time.
.png)
Global Platform for Preventative Care
As Ouva accumulates insights from the events that lead to patient deterioration in hospitals like Radboudumc, it is growing to be a platform for all facilities to benefit. The Ouva and Radboudumc teams are creating a global consortium where they can apply findings in one hospital across others that use Ouva to improve their patients' recovery.
With a diverse set of applications, Ouva quickly became a platform to help Radboudumc achieve quadruple-aim: Improving clinical experience, lowering costs, improving outcomes, and patient experience. Recognizing this potential, the team at Radboudumc is championing Ouva's expansion across the organization.
Get the Full Study
To get a copy of the complete study with 27 patients, physicians, nurses and researchers, follow the link below.
- Hospital-Acquired Conditions lead to avoidable cost and excess deaths, IBM Watson Health
- World Health Organization, Ageing and health
- Priscilla Holdren et al. “Burnout syndrome in hospital nurses”, Marshall University
- 2019 National Healthcare Retention & RN Staffing Report, Nursing Solutions, Inc.
- Corcoran JR, Herbsman JM, Bushnik T, Van Lew S, Stolfi A, Parkin K, McKenzie A, Hall GW, Joseph W, Whiteson J, Flanagan SR. Early Rehabilitation in the Medical and Surgical Intensive Care Units for Patients With and Without Mechanical Ventilation: An Interprofessional Performance Improvement Project. PM R. 2017 Feb;9(2):113-119. doi: 10.1016/j.pmrj.2016.06.015. Epub 2016 Jun 23. PMID: 27346093.
- Medical Costs of Fatal and Nonfatal Falls in Older Adults, Journal of the American Geriatrics Society
- Jeannie P. Cimiotti et al, “Nurse staffing, burnout, and health care–associated infection”, American Journal of Infection Control
- Renee Yao, NVIDIA Inception Partners Won Veterans Affairs AI Tech Sprint Awards with Latest AI Technologies
- Agostina J. Larrazabal, Nicolás Nieto, Victoria Peterson, Diego H. Milone, Enzo Ferrante, "Gender imbalance in medical imaging datasets produces biased classifiers for computer-aided diagnosis"
- Meg Tirrell, Leanne Miller, "Moderna slows coronavirus vaccine trial enrollment to ensure minority representation, CEO says"
- Weenk M, van Goor H, Frietman B, Engelen LJ, van Laarhoven CJ, Smit J, Bredie SJ, van de Belt TH. Continuous Monitoring of Vital Signs Using Wearable Devices on the General Ward: Pilot Study. JMIR Mhealth Uhealth. 2017 Jul 5;5(7):e91. doi: 10.2196/mhealth.7208. Erratum in: JMIR Mhealth Uhealth. 2021 Jul 16;9(7):e31899. PMID: 28679490; PMCID: PMC5517820.